DERMA-VISION
Skin diseases are among the most common human illnesses, affecting millions of people worldwide. The World Health Organization (WHO) estimates that skin conditions account for about 1.79% of the global disease burden. Early detection of skin diseases, particularly malignant conditions like melanoma, significantly improves prognosis and treatment outcomes. Early intervention can prevent the progression of skin ailments, reduce the severity of symptoms, and minimize the risk of complications. This underscores the importance of regular skin examinations and prompt medical attention for suspicious lesions. Computer-Aided Diagnosis (CAD) systems have emerged as valuable tools in dermatology, offering several benefits, including increased accuracy, efficiency, and standardization.
Whole Slide Imaging (WSI) digitizes entire tissue slides at high resolution, allowing for detailed examination and analysis of skin biopsies. WSI plays a crucial role in modern dermatopathology by enabling the digitization of entire tissue sections for detailed examination and archiving. Segmenting different skin layers in WSI is critical for diagnosing diseases, as precise segmentation helps in identifying pathological changes in specific skin layers, aiding in accurate diagnosis. Detailed analysis of skin layers provides insights into the pathophysiology of skin diseases, contributing to the development of new treatments.
Incremental Learning Framework
To automate the skin disease diagnosis procedure, we have proposed frameworks for layer segmentation and disease classification. AI models experience catastrophic forgetting when trained for new tasks. To address this challenge, we have employed increment learning framework, where we have in-cooperated a combination of loss functions (knowledge distillation, Kullback–Leibler, divergence loss, classification loss) in addition to cross entropy.
Two-dimensional hybrid incremental learning framework
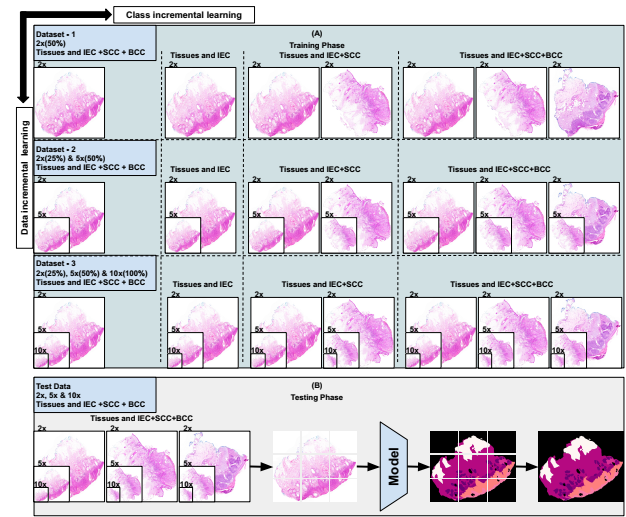
Two-dimensional hybrid incremental learning framework for accurate cancer segmentation and whole slide image analysis
To enhance the cancer segmentation from whole slide images, our proposed incremental learning framework for semantic segmentation, named 2-dimensional hybrid incremental learning (2DHIL), is a novel framework configured to train Transformer simultaneously in two directions as shown in Figure 2. The learning along the horizontal axis is class incremental learning and along the vertical axis, it is data incremental learning. To train the model we have utilized Queensland dataset, captured at three magnifications i.e., 10x, 5x, 2x annotated for 12 classes including skin layers (Epidermis, hypodermis, papillary dermis, keratin, reticular dermis), skin structures (Glands, Follicles, Inflammation) and skin cancer (Squamous cell carcinoma, Basal cell carcinoma, Intraepidermal carcinoma). Our training phase comprised of three straining stages, where each stage involved training model on a newly exposed class keeping a replay from previously learned classes.
Skin cancer classification using histopathology Images via incremental learning
Our proposed incremental learning framework is designed to classify non-melanoma skin cancer and it trains a model in two directions. Class incremental learning enhances the model’s capability and enables it to classify more classes after each incremental learning stage. Initially, the model is trained to identify IEC and SCC. Later, the training proceeds in two directions. Along the vertical axis, the model is trained to identify new classes whereas along the horizontal axis, it learns from a new dataset. In the next step, the model is incrementally trained to recognize BCC besides previously learned classes. This training phase involves presenting the model with training data and labels of the previous and new classes. The model trained using our incremental learning framework can classify more classes as compared to the original model. It is also capable of handling heterogeneous data from different datasets if trained with them incrementally. The final trained model can accurately classify non-melanoma skin cancer i.e., IEC, SCC, and BCC. This incremental learning framework enhances the robustness of the model, enabling it to adapt and improve over time.
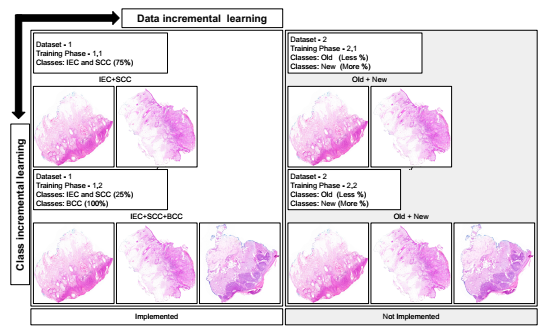
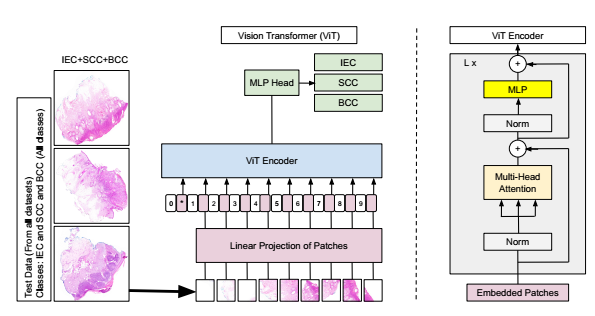
Incremental learning framework for Skin cancer classification using vision transformer
Relevant Publications
- Imran, Muhammad, Muhammad Usman Akram, Mohsin Islam Tiwana, Anum Abdul Salam, Taimur Hassan, and Danilo Greco. “Two-dimensional hybrid incremental learning (2DHIL) framework for semantic segmentation of skin tissues.” Image and Vision Computing 148 (2024): 105147.
- Imran, Muhammad, Mohsin Islam Tiwana, Mashood Mohammad Mohsan, Norah Saleh Alghamdi, and Muhammad Usman Akram. “Transformer-based framework for multi-class segmentation of skin cancer from histopathology images.” Frontiers in Medicine 11 (2024): 1380405.
- Asaf, Muhammad Zeeshan, Babar Rao, Muhammad Usman Akram, Sajid Gul Khawaja, Samavia Khan, Thu Minh Truong, Palveen Sekhon, Irfan J. Khan, and Muhammad Shahmir Abbasi. “Dual contrastive learning based image-to-image translation of unstained skin tissue into virtually stained H&E images.” Scientific Reports 14, no. 1 (2024): 2335.